SASSI
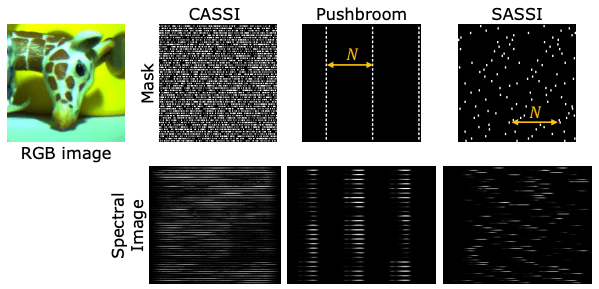
Video-rate Hypterspectral Imager
We introduce a novel video-rate hyperspectral imager with high spatial, temporal and spectral resolutions. Our key hypothesis is that spectral profiles of pixels within each super-pixel tend to be similar. Hence, a scene-adaptive spatial sampling of a hyperspectral scene, guided by its super-pixel segmented image, is capable of obtaining high-quality reconstructions. To achieve this, we acquire an RGB image of the scene, compute its super-pixels, from which we generate a spatial mask of locations where we measure high-resolution spectrum.
Super-Pixelated Adaptive Spatio-Spectral Imaging
The hyperspectral image is subsequently estimated by fusing the RGB image and the spectral measurements using a learnable guided filtering approach. Due to low computational complexity of the superpixel estimation step, our setup can capture hyperspectral images of the scenes with little overhead over traditional snapshot hyperspectral cameras, but with significantly higher spatial and spectral resolutions. We validate the proposed technique with extensive simulations as well as a lab prototype that measures hyperspectral video at a spatial resolution of 600 × 900 pixels, at a spectral resolution of 10 nm over visible wavebands, and achieving a frame rate at 18fps.
Compact
More Info
We provide a compact, light efficient design for the SASSI camera.
High Spatial Resolution
More Info
if we knew the regions with homogeneous spectra a priori, then we could sample one or more spectral profile for each region and propagate it to the remaining pixels, which would avoid blurring of the measurements as well as loss of spatial resolution.
Video-rate
More Info
Since all measurements being fused are captured simultaneously in a time-synchronized manner, we avoid the need for registration of images across time instants.
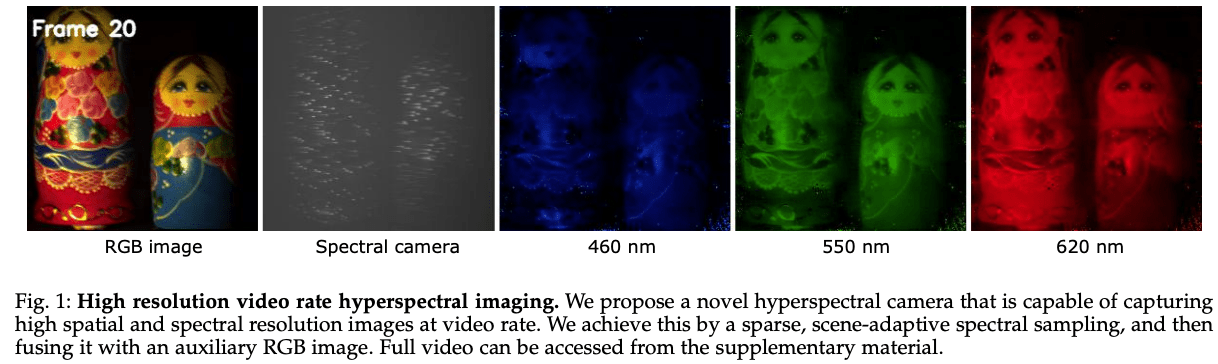
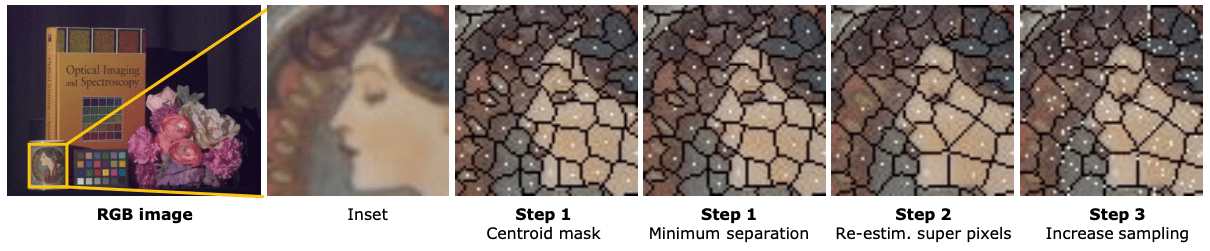
Super-pixel sampling strategy. We follow a computationally simple, three step sampling strategy for estimating the mask. Given a super-pixel segmentation, we first create a mask with centroids of super-pixels as sampling locations. Then, we enforce minimum separation along horizontal direction by moving/removing sampling locations. Next, we re-estimate the super-pixels with the new sampling locations as centroids. Finally, we increase light throughput by creating sampling locations everywhere with minimum separation between neighbors. Such a sampling strategy requires less than 2 ms on a modern computer, ensuring real time mask generation.
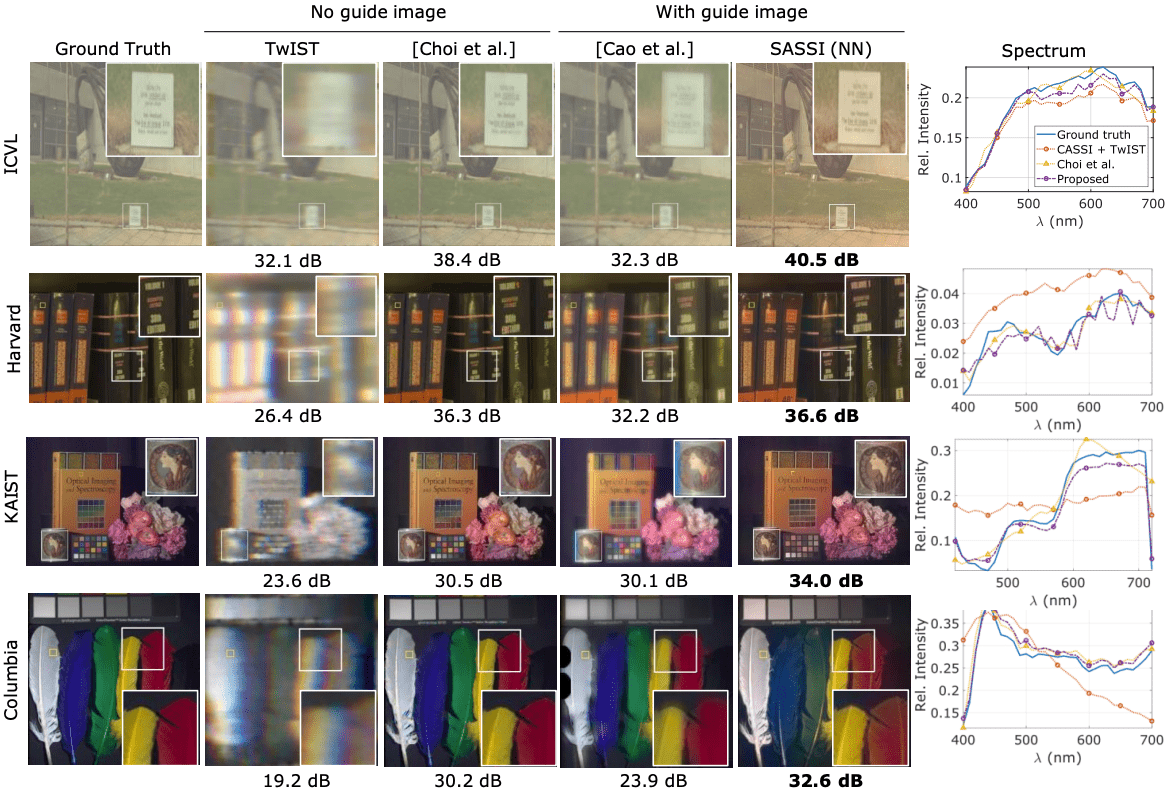
Comparisons with CASSI techniques. We compared reconstruction approaches which do not use a guide image, and ones that use guide image. SASSI outperforms other techniques across the board.
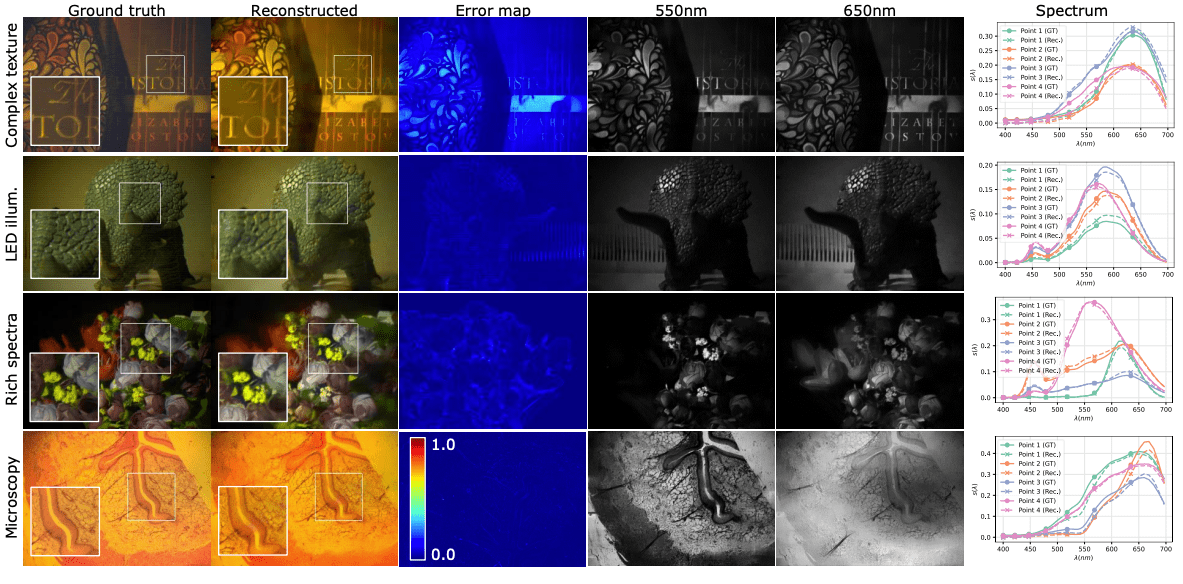
Real experiments. The figure showcases results across a variety of scenes with varying scene, spectrum, and illumination complexity. Across the board, our sampling and reconstruction approach produces accurate results with reconstruction accuracy exceeding 32dB.
People
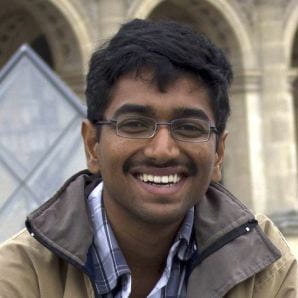
Vishwanath Saragadam
Michael DeZeeuw

Richard G. Baraniuk

Ashok Veeraraghavan

Aswin C. Sankaranarayanan